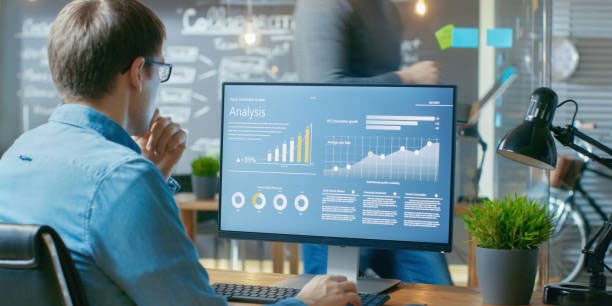
28 Oct AI Based Conversational Analytics | Future of Data
Human Race survives with meaningful conversations. Ability to converse and communicate is what sets us apart from other living beings. The way in which people interact with brands is continuously evolving and we are always looking at means to improve this interaction. Businesses are always competing to provide newer conversational experiences by leveraging Artificial Intelligence chatbot solutions for the enterprise and various voice enabled applications. . Wearable devices and internet of things are all intelligent outcomes of this effort to craft smarter conversations.
Businesses grow and flourish by taking a deeper look at what customers need. Conversational Analytics help businesses in this regard, and the main benefits of this technology include sentiment analysis, improved social listening and enhanced customization to better meet user needs.
What is Conversational Analytics?
An intelligent technology which transcribes speech and converts it into data using a transcription engine is called Conversational Analytics. This data is then structured so that conversations can be organised and analysed for deriving business insights. On the technical side, Conversational Analytics solutions contains an indexing layer which makes data searchable, along with a query and search user interface which lets the user describe requirements and perform high quality searches. Also, this contains simple reporting applications to present the analytics mainly in graphical form and numerous business applications to meet specific user requirements.
Conversational Analytics is specifically useful for user segmentation by identifying their needs and requirements and thus helps in providing the exact products/services which they are looking for. With this technology you can easily pull out a target demographic or a specific user to understand and analyse what they converse to a chatbot application with solid views of entire conversation as and when it happens. This throws light on what made the user leave the chatbot or voice application, this information is essential for user retention and for improving applications and better understanding your users.
An overview on Current Conversational Systems
Conversational systems use language as a medium to interact with customers. Microsoft research traverses dialogs that make use of language exclusively or in juxtapositions with extra modalities like gestures. Language can be spoken or text format and this is used in a varied setting such as conversational systems in devices and applications and established interactions in the real world.
Advancements in AI and Natural Language Processing (NLP) are the main factors which drive the rise of conversational analytics. This has specific applications in the field of robotics combined with computer vision. Technologies like Apple Siri, Microsoft Cortana, Amazon Alexa always help users to comfortably interact with their devices anywhere anytime. These technologies use natural language to make business interactions more meaningful and relevant. Alexa voice services enable businesses to integrate voice technology into their products. Alexa Skills Kit enables developers to design associated backend systems which would bring about meaningful conversations.
Capabilities of popular conversational systems are as follows:
- The user interfaces are human-centric and not machine-driven.
- The technology reproduces human-like conversations and help in understanding customer needs to help devise specific products/services.
- These systems are no longer limited by applications which run within them and capabilities always grow. Users once they get used to interacting with these systems need not go back to using those applications.
- Conversational analytics would transform businesses by providing an efficient way to interact with business data in an accessible system using natural language.
Conversational AI Maturity Levels
Conversational AI is a known concept and it is gaining great popularity in recent years. Tech Titans like Google repeatedly affirm that we are moving from a mobile-first to an AI-first world. Users expect technology to be meticulously contextual, intuitively conversational and evolutionarily proficient.
AI chatbots are designed to handle customer questions and respond with pre-built responses based on rule-based conversation processing. Contextual assistants surpass replying to simple questions or sending push notifications. Let us have a look at Maturity Levels of Conversational AI:
Level 1 Maturity: At this level chatbot performs the basic customer support actions such as sending notifications/reminders or responding to questions. For example, chatbot would provide you a link to locate a specific place of your interest.
Level 2 Maturity: At this level, chatbot replies to a FAQ and does a simple follow-up process which would help you with cross-selling. For instance, along with providing a link to locate a place, it would suggest nearby dining options.
Level 3 Maturity: At this level, virtual assistant understands the context of conversation and responds to unexpected questions. For example, the travel bot which shows you quite a few vacation spots and arranges easy and secure travel.
Level 4 Maturity: At this level, the contextual assistant understands your preferences and offers customised recommendations for proactive customer handling.
Level 5 Maturity: At this level, the contextual assistants would monitor and manage a group of other assistants which would help in operations. For example, they would plan promotions on travel experiences, target specific customer segments to increase conversion rates etc.
Role of NLP in AI based Conversational Analytics
Computers need to process and understand human language to make good business decisions. Natural Language Processing (NLP) is an application of Artificial Intelligence solutions which makes this possible. Advancements in Machine Learning and Deep Learning have also helped enormously in this area. Deep learning models analyse huge volumes of text and delivers text summarization, language translation, sentiment analysis and context modelling.
Natural Language Understanding (NLU) which is a subset of NLP converts natural language into structured data. NLU can do intent classification and entity extraction which are the key drivers of conversational AI. Intent means something which a user is trying to convey. Intent classification is done by first feeding a NLU model with labeled data which provides a list of known intents and sample sentences corresponding to those intents. This model once trained would classify a new sentence which it sees into a predefined intent. Entity extraction is the act of identifying key pieces of information in a specific text.
How to train a Conversational AI
Conversational AI can help your business scale quickly if trained in the right way. Training a conversational AI chatbot is intuitive most of the times. Simply put, a frequent question is chosen and chatbot is trained to identify it and then creates the answer. Once the answer is set live the bot would reply to all customers who ask a similar question. You can save a lot of time by creating more answers.
Following are the main steps in training a conversational AI:
- Training to Recognize the question: Conversational AI can identify common questions from the conversation history, and you must make sure it identifies all possible variations of those questions. So, this must be discussed with teams to recognize the different ways in which the same question can be asked.
- Crafting replies for a wide range of topics: Customers will have numerous questions and replies can be crafted for common topics. This provides instant resolutions most of the times.
- Include several questions on the same topic: Customers ask different questions for each individual topic. For example, if your topic is directory, the common question list would be:
- Where do I view the directory?
- How do I find a specific contact in it?
- How do I locate the contact?
Each of these questions are related to the single topic but they require distinct and several answers. Conversational AI can understand the gradations between different questions within the single topic by creating many answers. So, most of the times it delivers precise answers.
Future of Conversational Analytics
Data is much richer and greatly actionable these days with the advancement of technology. Conversational interfaces which are Text based like Facebook or Slack and those which are voice based like Alexa or Google Home are getting more and more popular. Connected cars, connected homes and highly interactive devices are going to be the innovative game changers in the coming years. Systems and devices which respond to customer queries based on customer mood and sentiments would dramatically change the way businesses communicate with customers.
Users have the freedom to send and receive data in the form of their own words, voices and images and get the relevant replies from conversational AI systems. It also provides much advanced actionable insights such as introducing a live person whenever necessary or sending push notifications based on the specific customer segment.
Conversational data is the future mainly because:
- It helps you to identify patterns in user interactions, the way they interact with chatbots or any device which has a conversational interface.
- It shows details of user interactions in real time
- Interaction records can be stored to devise retention strategies and better applications.
The more you understand your customers using a thorough analysis of their interests during the conversation, the better would be your strategies and profitability.
Get in touch to know more.